Data Science: a fresh wind through genomics, physics and history
Text: Jorn Lelong
There are few university researchers who get as much interaction with other fields of science as the data scientists of the CIT, the Centre for Information Technology of the University of Groningen.
Apart from training in Data Science at the IT Academy Noord-Nederland, Campus Fryslan and CBS, the team is involved in 30 data projects for a wide array of departments - including genomics, physics and history.
Promising projects
The Data Science team was brought to life in November 2016, on the initiative of the IT Strategy Committee of the RUG. Jonas Bulthuis, at the time consultant for the department of Research and development, had noticed data science was becoming a trend and took the lead. With the initial budget, they managed to hire two data scientists and they started executing some promising projects.
Call for proposals
Since then, the Data Science has launched a yearly call for proposals, for which researchers from all faculties can propose a research topic involving data science. As more and more researchers are discovering the possibilities that data science can offer, the demand is growing, says Dimitrios Soudis, one of the CIT’s data scientists. “For the first call, we received 14 proposals. The year after we got close to 40. So you see more people are approaching us and saying: what could you do for us?”

Team of ten people
When Dimitrios started working for the CIT, some 1,5 years ago, the Data Science team consisted of just three people. By now they’re ten - each with their own expertise. “Since we’re a relatively small team, it’s crucial we complete each other”, says Jonas Bulthuis.
That’s why within the Data Science Team, you’ll find people with a background in econometrics, physics or math. When they assign projects, they try to take everyone’s specialty and interest into account. “We want to have people working on projects they are truly passionate about. It’s much more motivating.”
KVI: A deep dive into particle physics
Taking data scientists’ interests into account is exactly what happened when they assigned the project for KVI-CART, the Center for Advanced Radiation Technology. Due to their affinity with the subject, it was assigned to Cristian Marocico and Leslie Zwerver. Cristian for example has a degree in physics himself, which proved to be very useful, says Johan Messchendorp, nuclear physicist and promoter of this project: “As a physicist you have certain expectations, which helps you look for the relevant information. Or when I’m talking about the conservation of energy or impulses, he instantly knows what I’m getting at.”
Subatomic particles
For a few years now, Johan Messchendorp has been focusing on particle physics, a field within physics that looks into subatomic particles, like protons and neutrons. These subatomic particles are only visible through high-speed collisions in particle accelerators. But as particle accelerators enable an increasing number of collisions per second, the amount of data expands equally. Therefore, conventional research methods meet their limits. “So it’s our task to come up with a smart solution”, explains Johan Messchendorp.

Expose patterns
That’s why he reached out to the Data Science team. With machine learning, a method of AI that allows to reveal patterns, data scientists Leslie and Cristian try to filter relevant bits from the redundant information: background reduction, as it’s called. The whole process needs to be done online, since no computer is capable of storing the tons of data they work with.
Needle in a haystack
This machine learning will eventually help to reconstruct gluons, nuclear particles that carry lots of information, but that are equally hard to see. In fact, they only appear in combination with other particles. That’s why Messchendorp wants to collide protons with antiprotons in order to create glueballs, exotic states that contain gluons. Still, it’s looking for a needle in a haystack, as such exotic states only appear once in a 100 billion collisions.
Positive results
The research into gluons cannot yet be started, since the particle accelerator that allows such high-speed collisions is still being built in Darmstad, Germany. Therefore Leslie and Cristian are currently working with data extracted from a particle accelerator in China, with much less collisions. “These data are still manageable, and allow us to optimize the technique and compare the results with conventional methods”, Messchendorp explains. The first signs look promising. In just a few months, Leslie and Cristian have developed an algrithm that stands the test of comparison against classical methods.
New wind
According to Messchendorp, these kinds of comparative tests are essential to breathe new life into physics. “Our community likes to stick to familiar methods. So if you come up with something new, you quickly get asked: are you sure this might work? Therefore you need to prove it works with existing data, before applying it to more complex structures.”
GCC: A new screening tool for hereditary diseases
Dimitrios Soudis, data scientist at the CIT, also recognizes the academic world often clings to its conventional methods: “Academics mainly want to publish in journals. But if the journals are not open to new techniques, the researcher waits too. Therefore it takes a while until a new techniques spills over to another faculty.
Genome of the Netherlands
The Genomics Coordination Centre of the RUG is an exception in this regard. For more than ten years, the department has been doing groundbreaking data research, such as the national project Genome of the Netherlands in 2014.
Still, there is a big difference between data gathering, statistical modelling and more advanced machine learning. “Through our experience, we’re quite good at classifying genomic variants as benign or pathogenic or unknown. But the question was: can we now predict for a new genomic variant what the effect will be?”, explains Joeri van der Velde, researcher of the GCC.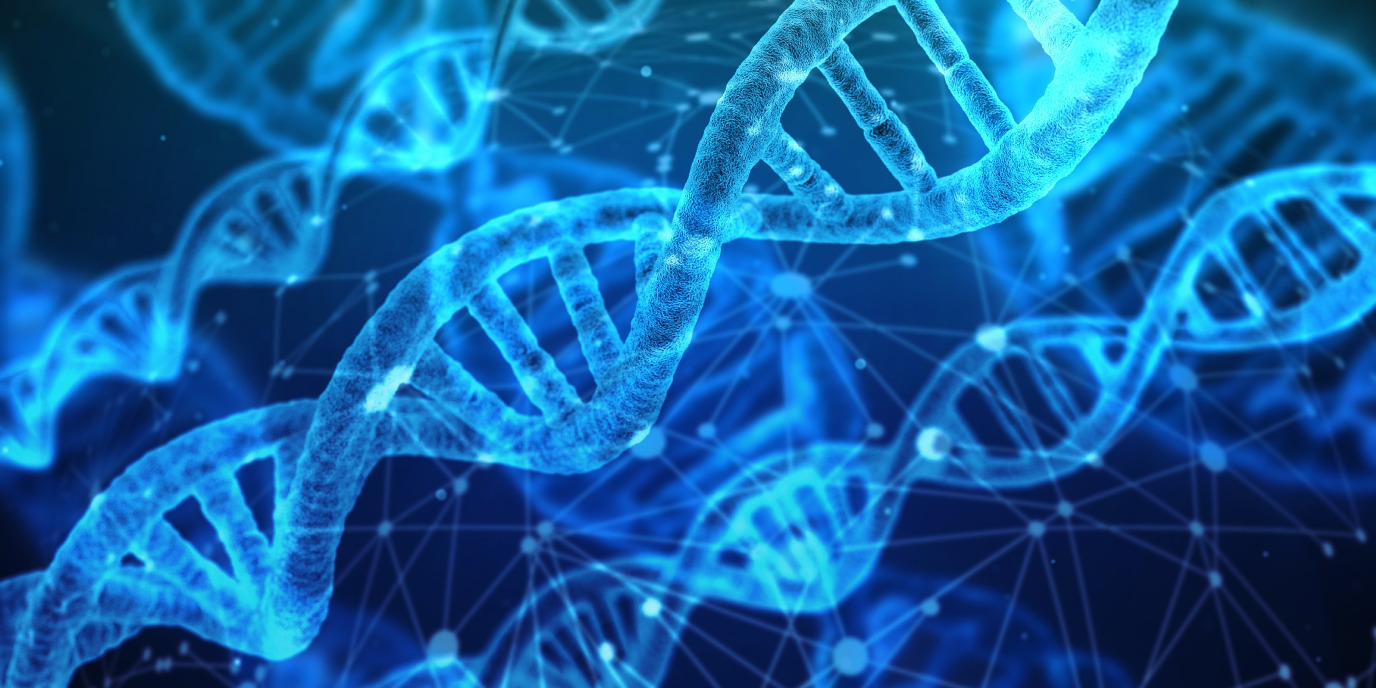
New machine learning model
Together with PHD student Li Shuang and Dimitrios Soudis, he tackled this question. The goal was to modify the existing CADD model, which is used worldwide to annotate genetic mutations. “But instead of modifying CADD, we used the information behind it and passed it into a machine learning model. In fact, we reinvented the model”, says Dimitrios Soudis.
Dimitrios and his team gave themselves a week. But soon followed very promising results; which they immediately passed on to Joeri and Shuang. “For two weeks I was really paranoid”, admits Dimitrios. “I said to everyone: don’t be excited, there is probabaly an error somewhere. But when we applied our model to new data, it seemed to perform as well. “I thought: how come no one has tried this before?”
Added value
Joeri van der Velde was equally surprised by the quick results. “The tool already outperforms CADD and other methods greatly in the questions that we want to address.” According to him, data scientists make the difference when it comes to applying the maching learning models: “I always thought building the algorithm was the most difficult part. But I was surprised how difficult it is to apply the model in order to tackle te research question in the best way. That is an expertise on its own.”
Unknown gene variant
The algorithm they created is already capable of distinguishing pathogenic genes from benign genes. Moreover, it allows them to link these variants with genomic features and predict if unknown genes within the DNA are pathogenic.
Predicting hereditary diseases
Their results will now be passed on to lab specialists. Then, researchers can develop it into an effective screening tool which can be used in the doctor’s office. It’s these close ties with the practice of medicine that make this project rewarding, says Joerie van de Velde. “Through this project we eventually ail to diagnose and predict hereditary diseases much quicker. That is a big breakthrough in our field.”
Similarly, Dimitrios Soudis is excited about this project. “As a statistician, you get to play in everyone’s backyard, said John Tuckey. And it’s true: I’ve been involved with many different projects, and all were interesting in their own way. Still, I have developed a preference for the medical field, since I feel like I can make a real impact here.”
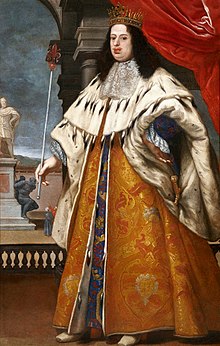
History: A virtual journey to the 17th century Netherlands
Not only beta scientists and the medical field are calling upon the expertise of RUG’s data scientists. At the Faculty of Arts, there is growing awareness of the possibilities of data science to complete classical research methods. Sabrina Corbellini, professor of Medieval History, for example, does innovative research on the travels of Cosimo III dè Medici. As a member of the glorious banking family and dynasty of Medici, he became the Duke of Florence at the end of the 17th century.
Italian fascination for the Netherlands
For this project, Sabrina Corbellini focuses on his travels to the Netherlands, between 1667 and 1669. Cosimo thought very highly of the Dutch. He carefully took notes on how the Dutch cultivated their landscape with water management and windmills. Moreover, he tried try to apply the form of government of the Dutch East India Company when he came into power in Florence.
Diaries
According to Corbellini, the different maps and diaries, which were kept for ages in the library of the Medici, contain a wealth of information on his travels. “Cosimo visited art dealers, writers and all kinds of collectors. He went to different cities and spoke to mayors. He wanted to know everything that was going on. And all that can be read in his diaries, from hour to hour.”
Through these diaries, Corbellini wants to trace where Cosimo went, whom he got into touch with and for what reason. But reading and analysing old-Italian diaries is very time-consuming. Therefore she asked data scientists Venustiano Soancatl Aguilar en Nicoletta Giudice to write a script which allows them to label locations, persons and dates in the diaries.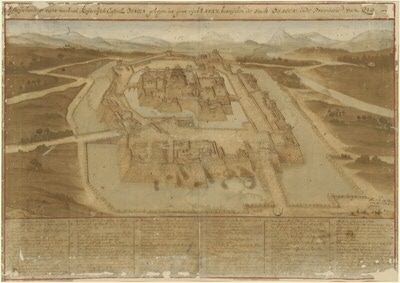
Language is more complicated
For Venustiano Soancatl Aguilar, it’s the first time he’s involved in historical research. “Earlier I have been working on projects with real-time data on body movement, or projects in astronomy. There, I worked with much larger datasets. However, that doesn’t mean this project is easier. Numbers are universal, they always mean the same. But working with language is perhaps the most complicated, as one word can have several meanings.”
Optimize model
Moreover, the diaries are written in Old-Italian, which doesn’t make it easier. Luckily he can count on his Italian colleague Nicoletta Giudice for help. “Machine learning alone won’t tell you if you make mistakes. That’s why Nicoletta checks and gives feedback, which we can use to train the model”, Venustiano explains. Indeed, optimizing the model is the hardest part. But in the long run, machine learning saves time, thinks Venustiano. “Once the model works, you can easily apply it to new documents. That’s a lot faster than analysing 100 diaries manually.”
Expertise
It’s the first time Sabrina Corbellini is working with data scientists for a history project. Yet, she plans on maintaining close ties. “In the projects I am working on now, I focus a lot on spaces where knowledge is transmitted, such as hospitals, pharmacies or universities. And when it comes to geolocalisation, data scientists have the expertise that classical historians are lacking. We need to get to know each other better, so we learn how we can complete each other.”Last modified: | 04 October 2024 12.16 p.m. |
More news
-
10 June 2024
Swarming around a skyscraper
Every two weeks, UG Makers puts the spotlight on a researcher who has created something tangible, ranging from homemade measuring equipment for academic research to small or larger products that can change our daily lives. That is how UG...
-
21 May 2024
Results of 2024 University elections
The votes have been counted and the results of the University elections are in!